In today’s rapidly advancing technological landscape, the demand for compact and lightweight AI and machine learning capabilities for embedded systems is soaring. Industries ranging from aerospace to consumer electronics are increasingly relying on sophisticated algorithms and intelligent features that need to fit into smaller, more efficient packages. Whether it’s a drone navigating autonomously or a wearable device providing real-time health analytics, the integration of AI and machine learning into embedded systems is revolutionizing the way we interact with technology.
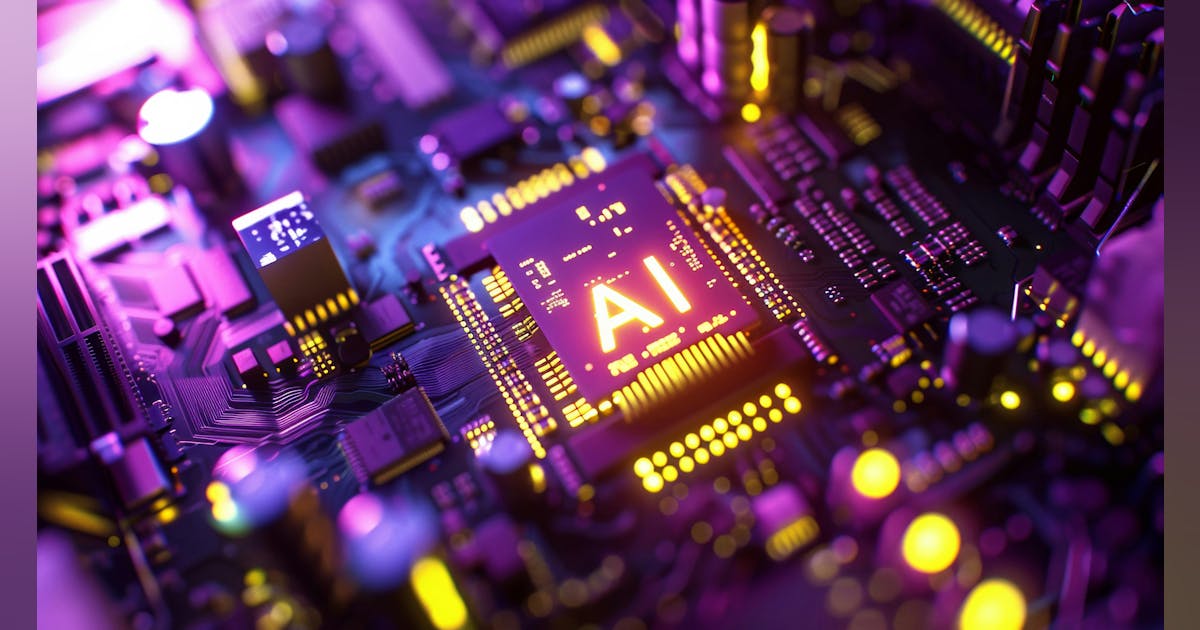
*Image Source: Military Aerospace*
However, this rapid shift towards smarter, more connected devices brings new challenges. Designing compact and lightweight AI solutions is no easy feat. Developers often find themselves struggling to balance power efficiency with processing capabilities. Limited space for hardware, heat dissipation issues, and the need for robust, real-time processing are just a few hurdles they must overcome. Additionally, there is a growing necessity for low-latency and reliable performance, which is paramount in mission-critical applications such as aerospace and defense.
Why is achieving this balance so crucial? Imagine a scenario where an autonomous vehicle’s AI system lags or fails due to hardware constraints. The consequences could be catastrophic. Therefore, the question isn’t just about miniaturizing the technology but about maintaining its reliability and performance.
For those targeting the development of such embedded AI systems, the goal is quite clear: create hardware that supports extensive AI and machine learning functionalities without compromising on efficiency or reliability. This means investing in advanced materials, innovative design architectures, and powerful, yet efficient, processing units. Moreover, software optimization plays a crucial role in ensuring that the AI algorithms run smoothly on these constrained platforms. Industries are looking for solutions that not only deliver top-notch performance but also fit within stringent size, weight, and power (SWaP) limitations.
In summary, as we progress towards an era where AI and machine learning are integral to embedded systems, the need for compact and lightweight solutions becomes increasingly critical. The future of intelligent devices hinges on overcoming these engineering challenges to provide seamless and reliable experiences in even the most demanding environments. Whether it’s the aerospace sector requiring robust systems or consumer electronics focusing on convenience and usability, finding the right balance between capability and compactness is key to unlocking the full potential of embedded AI.